Despite the hype around AI, many ML projects fail, with only 15% of businesses' ML projects succeeding, according to McKinsey. Particularly with the significant investments in large language models and generative AI, only a small portion of companies have managed to realize their true value.
Drawing from her 10 years of experience at the forefront of bringing ML to production at various companies, Wenjie will explore common pitfalls that cause these failures, such as the inherent uncertainty of machine learning, misaligned optimization objectives, and skill gaps among practitioners. She will also highlight critical factors companies must consider when shaping and developing their ML strategies and execution plans. Attendees will gain actionable insights to increase their chances of success in the ever-evolving landscape of machine learning.
Main Takeaways:
- Walk through common pitfalls in ML projects: misaligned objectives, skill gaps, and inherent uncertainty.
- Share experiences for performance monitoring, continuous skill development, and effective team collaboration.
- Understanding the important factors to help develop AI or GenAI strategies can help harness the true value of machine learning and achieve tangible business outcomes.
Speaker
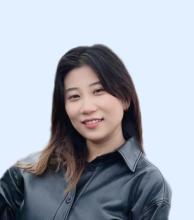
Wenjie Zi
Senior Machine Learning Engineer and Tech Lead @Grammarly, Specializing in Natural Language Processing, 10+ Years of Industrial Experience in Artificial Intelligence Applications
Wenjie Zi holds a Master’s degree in Computer Science from the University of Toronto, specializing in Natural Language Processing (NLP). Currently, she serves as a Senior Applied AI Engineer and Technical Lead at Grammarly, bringing over ten years of industrial experience in artificial intelligence applications. Wenjie has successfully implemented and deployed various projects, including Retrieval Augmented Generation (RAG), recommendation systems, semantic parsing (natural language to SQL), and quantitative trading.
Her research has been published in leading conferences and workshops such as ACL, NeurIPS, and KDD. Additionally, Wenjie is a course instructor for the certificate program at the University of Toronto, where she teaches deep learning-related subjects.
In her spare time, Wenjie actively participates in the Canadian AI community, serving as a committee member of MLOps World and as the lead of the Women in AI Canada Sponsorship team. She co-founded the Toronto AI Practitioners Network (TAPNET) in early 2024 and has organized multiple meetups with over 100 participants, aiming to connect technology practitioners across North America.