Recommendation and search systems are two of the key applications of machine learning models in industry. Current state of the art approaches have evolved from tree based ensembles models to large deep learning models within the last few years. This brings several modeling, systems, infrastructural and software challenges and improvements with it. Additionally, Large Language Models and Foundation Models are also rapidly starting to influence the capabilities of these real world search and recommender systems.
In this talk, Moumita will present an overview of industry search and recommendations systems, go into modeling choices, data requirements and infrastructural requirements, while highlighting challenges typically faced for each and ways to overcome them.
Speaker
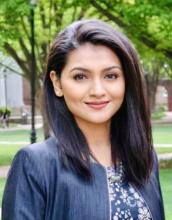
Moumita Bhattacharya
Senior Research Scientist @Netflix, Previously @Etsy, Specialized in Machine Learning, Deep Learning, Big Data, Scala, Tensorflow, and Python
Moumita Bhattacharya is a senior Research Scientist at Netflix, where she works on developing at-scale machine learning models for Search and Recommendation Systems. Prior to Netflix, she was a Senior Applied Scientist at Etsy, where she was tech leading a team that developed recommendation systems to show relevant products to Etsy users. Moumita has a PhD in Computer Science with a focus on Machine Learning and its applications. She has been actively serving as Program Committees for WebConf and is a reviewer for conferences such as RecSys, SIGIR, WebConf, AAAI, and various journals. Moumita is also an adjunct faculty in the Data Science Institute (DSI) of the University of Delaware .