Abstract
Ray is an open source project that makes it simple to scale any compute-intensive Python workload. Industry leaders like Uber, Shopify, Spotify are building their next generation ML platforms on top of Ray. Ray is equipped with a powerful distributed scheduling mechanism which launches stateful Actors and stateless Tasks in a much more granular and lightweight fashion than existing frameworks. Meanwhile it also has an embedded distributed in-memory object store to drastically reduce data exchange overhead. These architectural advantages make Ray the ideal compute substrate for cutting-edge ML use cases including Graph Neural Networks, Online Learning, Reinforcement Learning, and so forth.
This talk will introduce the basic API and architectural concepts of Ray, as well as diving deeper into some of its innovative ML use cases.
Speaker
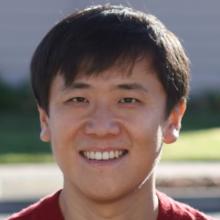
Zhe Zhang
Head of Open Source Engineering @anyscalecompute, Previously Hadoop/Spark infra Team Manager @LinkedIn
Zhe is currently Head of Open Source Engineering (Ray.io project) at Anyscale. Before Anyscale, Zhe spent 4.5 years at LinkedIn where he managed the Hadoop/Spark infra team. Zhe has been working on open source for about 10 years; he's a committer and PMC member of the Apache Hadoop project, and a member of the Apache Software Foundation.