Abstract
Building LLM-powered applications using APIs alone poses significant challenges for enterprises. These challenges include data fragmentation, the absence of a shared business vocabulary, privacy concerns regarding data, and diverse objectives among data and ML users.
In this presentation, we will share our approach to overcoming these hurdles by establishing a robust data foundation. We achieve this through the creation of an enterprise data fabric using knowledge graphs, adapting domain knowledge and vocabulary, and implementing data contracts for enhanced data observability. Leveraging this strong foundation, we have successfully accelerated the utilization of large language models, offering valuable solutions tailored to the specific needs of our customers in the supply chain industry.
Our solutions encompass a range of scenarios, such as:
- Risk mitigation: Identifying new suppliers in response to geopolitical risks, pandemics, inflation, and other factors.
- Environmental, Social, and Governance (ESG) framework implementation to achieve sustainability goals.
- Strategic procurement.
- Spend analytics.
- Data compliance.
Interview:
What's the focus of your work these days?
My daily routine typically includes:
Developing and managing a data and machine learning infrastructure, comprising multiple layers of machine learning and the latest advancements in large language models.
It also includes engaging in strategic discussions about the future of artificial intelligence and how we can create something valuable, reliable and safe for our customers (enterprises) while leading them through this Generative AI movement.
What's the motivation for your talk at QCon San Francisco 2023?
Innovation in AI is often associated with tech giants like Google, OpenAI, Doordash, Shopify, Spotify, Meta, Netflix, and others. These companies are well-prepared for such advancements, and their innovation doesn't come as a surprise.
However, large enterprises operating in the global market face unique challenges. They span diverse industries and encounter difficulties in product and service delivery while safeguarding customer data privacy and security. Moreover, the need to empower an aging workforce with technology further adds complexity. Therefore, implementing machine learning models requires careful consideration of technology enablement, design, and data privacy.
While the technology landscape evolves rapidly, it remains crucial for engineers to dedicate time to developing meaningful and valuable products that meet customer needs. This talk aims to showcase how large enterprises can accomplish this feat and invites dialogue to learn from others' perspectives and experiences in implementing similar systems.
How would you describe your main persona and target audience for this session?
- Directors of Data/ ML , Data and ML architects
- Data scientist
- ML engineers
- Data engineers
- Product Managers / UX Designers.
Is there anything specific that you'd like people to walk away with after watching your session?
- Why is it crucial to make a substantial investment in establishing a strong foundation for data and machine learning?
- What are the potential Economies of Machine Learning that come into play?
- What is the concept of enterprise data fabric, and how can it be achieved using Knowledge graphs?
- How can one harness the capabilities of large language models in conjunction with Knowledge graphs?
- What does the future hold for developing a domain-specific co-pilot using Federated agents?
Speaker
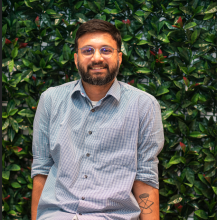
Nischal HP
Vice President of Data Science @Scoutbee, Decade of Experience Building Enterprise AI
Nischal, a data science and engineering leader with over 13 years of experience in Fortune 500 companies and startups, is renowned for his visionary approach in leveraging machine learning to drive product advancements. He excels at building and leading teams while fostering a culture of innovation and excellence. Nischal's international exposure and cross-industry expertise further enrich his ability to create impactful solutions in the dynamic field of data science.