In the rapidly evolving digital landscape, the way we approach data architecture is undergoing a transformative shift. This shift is not just about adopting new technologies but about fundamentally rethinking our approach to data management, governance and architecture design. Welcome to the concept of "Shift-Left Data Architecture" – a methodology that promises to set the foundation for future-ready data ecosystems.
As data's role in decision-making, operations and machine learning has become increasingly critical, the need for a more proactive approach has become evident. We need to reconsider traditional methods where data considerations, and supporting ML often came later in the development process, which led to inefficiencies, increased costs, and data quality and outcomes issues. By shifting left, organizations can avoid costly revisions, enhance data security, and ensure that their data architecture is robust and scalable.
Join us to learn more about this new era in data architectures, the building blocks of a shift-left architecture, the tools and technologies that enable it, and gain insights on how to implement these principles effectively within your organization.
From this track
Beyond Durability: Enhancing Database Resilience and Reducing the Entropy Using Write-Ahead Logging at Netflix
Tuesday Nov 19 / 10:35AM PST
In modern database systems, durability guarantees are crucial but often insufficient in scenarios involving extended system outages or data corruption.
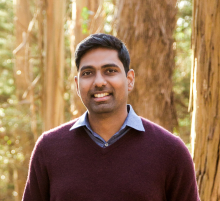
Prudhviraj Karumanchi
Staff Software Engineer at Data Platform @Netflix, Building Large-Scale Distributed Storage Systems and Cloud Services, Previously @Oracle, @NetApp, and @EMC/Dell
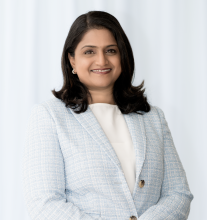
Vidhya Arvind
Staff Software Engineer @Netflix Data Platform, Founding Member of Data Abstractions at Netflix, Previously @Box and @Verizon
OpenSearch Cluster Topologies for Cost-Saving Autoscaling
Tuesday Nov 19 / 11:45AM PST
The indexing rates of many clusters follow some sort of fluctuating pattern - be it day/night, weekday/weekend, or any sort of duality when the cluster changes from being active to less active. In these cases how does one scale the cluster?
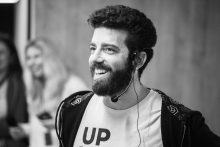
Amitai Stern
Engineering Manager @Logz.io, Managing Observability Data Storage of Petabyte Scale, OpenSearch Leadership Committee Member and Contributor
Stream All the Things — Patterns of Effective Data Stream Processing
Tuesday Nov 19 / 01:35PM PST
Data streaming is a really difficult problem. Despite 10+ years of attempting to simplify it, teams building real-time data pipelines can spend up to 80% of their time optimizing it or fixing downstream output by handling bad data at the lake.
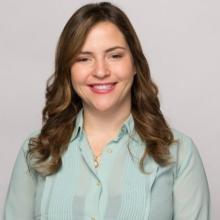
Adi Polak
Director, Advocacy and Developer Experience Engineering @Confluent
Stream and Batch Processing Convergence in Apache Flink
Tuesday Nov 19 / 02:45PM PST
The idea of executing streaming and batch jobs with one engine has been there for a while. People always say batch is a special case of streaming. Conceptually, it is.
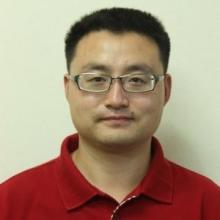
Jiangjie (Becket) Qin
Principal Staff Software Engineer @LinkedIn, Data Infra Engineer, PMC Member of Apache Kafka & Apache Flink, Previously @Alibaba and @IBM
Efficient Incremental Processing with Netflix Maestro and Apache Iceberg
Tuesday Nov 19 / 03:55PM PST
Incremental processing, an approach that processes only new or updated data in workflows, substantially reduces compute resource costs and execution time, leading to fewer potential failures and less need for manual intervention.
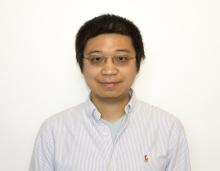
Jun He
Staff Software Engineer @Netflix, Managing and Automating Large-Scale Data/ML Workflows, Previously @Airbnb and @Hulu
Unconference: Shift-Left Data Architecture
Tuesday Nov 19 / 05:05PM PST