Abstract
The future of search is undergoing a revolutionary transformation, shifting from traditional linear queries to a rich multiverse of possibilities powered by AI. The talk explores three critical domains reshaping search experience: multimodal interactions, personalization through large user sequence; and the emerging role of AI agents for application simulation. Through production infrastructure examples and performance metrics across leading LLMs, this session examines the practical implementation challenges and solutions. The discussion concludes with insights into 2025+ market dynamics, including the emergence of agent-first search platforms and the impact of on-device intelligence on traditional search business models.
Speaker
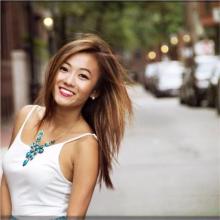
Faye Zhang
Staff Software Engineer @Pinterest, Tech Lead on GenAI Search Traffic Projects, Speaker, Expert in AI/ML with a Strong Background in Large Distributed System
Faye is a Staff Software Engineer at Pinterest, where she leads AI-driven search traffic initiatives and launched the company's first successful GenAI production experiment, driving significant user engagement growth. With a Computer Science degree from Georgia Tech and ongoing AI graduate studies at Stanford, she combines deep technical expertise in distributed systems with cutting-edge AI research. Her work spans both industry and academia, including contributions to university genomic science research. She regularly shares insights on AI innovation at technical conferences in San Francisco and Paris, focusing on scalable AI solutions that transform user experiences.