Running LLMs requires significant computational power, which scales with model size and context length. We will discuss strategies for fitting models to various hardware configurations and share techniques for optimizing inference latency and throughput at Meta.
As we transition from stand-alone LLMs to production grade systems that support LLM at a global scale, we delve into our approach to constructing systems that accommodate dynamic user requests and widespread product adoption. This includes implementing caching strategies and addressing infra latency, efficiency and reliability issues within real data centers of a heterogeneous hardware fleet.
Finally, we will present case studies that demonstrate our methods for achieving a balance between model quality, latency, throughput, reliability, and cost in a complex and demanding environment.
Speaker
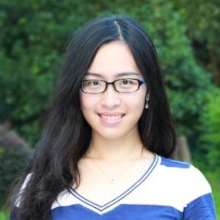
Charlotte Qi
Senior Staff Engineer @Meta
Ye (Charlotte) Qi is a production engineer on the AI inference team at Meta. She is one of the inference technical leads behind Meta’s initial Meta.AI product launch and LLaMa3 development.
With over six years of experience at Meta, she has run large-scale online inference systems for both RecSys and LLM models across various organizations. Charlotte enjoys working at the multidisciplinary intersection of infrastructure, machine learning, product development and DevOps, advancing end-to-end development from research to production. Her background spans the entire software stack, including hardware productionization, inference runtime optimizations, distributed system reliability, experiment management, and service operations.
Prior to joining Meta, Charlotte earned her Master's degree from Carnegie Mellon University, specializing in large-scale machine learning systems and neural machine translation.