Streaming databases have gained significant attention in recent years. From its name, it is evident that a streaming database combines the power of stream processing and databases. The fundamental concept behind streaming databases is to provide efficient data storage capabilities to facilitate seamless stream processing.
In this presentation, I will delve into the evolution of streaming databases over the past two decades. Furthermore, I will highlight the distinctive features and design principles that set streaming databases apart from conventional database systems and stream processing engines. Through the use of real-world scenarios, I will demonstrate how streaming databases can simplify the data stack and enhance cost efficiency when building real-time applications, such as network monitoring, ad recommendation, and crypto trading.
Interview:
What's the focus of your work these days?
I'm the founder of RisingWave Labs, the company that develops a distributed SQL streaming database called RisingWave. Our goal is to make stream processing simpler and more cost efficient.
What's the motivation for your talk at QCon San Francisco 2023?
Streaming databases are gaining popularity these days, but many people wonder how they differ from conventional stream processing systems and real-time OLAP databases. Starting with the basic concept, I will delve into technical details and use cases, explaining why people need streaming databases in their modern data stack.
How would you describe your main persona and target audience for this session?
My primary audience consists of data engineers or engineering leaders seeking modern technologies for real-time analytics and stream processing.
Is there anything specific that you'd like people to walk away with after watching your session?
I aim to provide my audience with a comprehensive understanding of the modern real-time data processing ecosystem, empowering them to make informed decisions when choosing among various systems.
Speaker
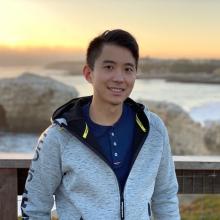
Yingjun Wu
Founder and CEO @RisingWave Labs, Previously Engineer @AWS Redshift & Researcher @IBM Research Almaden
Yingjun Wu is the founder of RisingWave Labs, a database company developing RisingWave, a distributed SQL database for stream processing. Before running the company, Yingjun was a software engineer at the Redshift team, Amazon Web Services, and a researcher at the Database group, IBM Almaden Research Center. Yingjun received his PhD degree from National University of Singapore, and was a visiting PhD at Carnegie Mellon University. He has been working in the field of stream processing and database systems for over a decade.